Data is the new oil. It fuels business growth, drives decision-making, and propels innovation.
But harnessing the power of data is no easy task. It requires specialized skills, advanced tools, and a deep understanding of data science.
Enter the data scientist.
These professionals are the wizards of the data world. They can turn raw data into crucial knowledge that will help you make smarter decisions and gain a competitive edge.
But how do you know when it’s time to hire a data scientist?
In this article, we’ll explore five critical signs that your business needs a data scientist now. We’ll delve into the challenges and opportunities that each sign presents, and how a data scientist can help you navigate them.
So, let’s dive in and discover the signs that it’s time to hire a data scientist.
Role of a Data Scientist: What Is It About
Data scientists are the architects of data-driven solutions. Their role involves using analytical, statistical, and programming skills to interpret complex data sets.
Think of them as detectives who solve business mysteries using data. They identify patterns, predict trends, and build models that uncover invaluable insights.
Businesses across sectors are increasingly relying on data scientists. From improving customer experience to optimizing operations, their applications are diverse.
Here are some key roles a data scientist typically performs:
- Data collection and cleaning: Gathering and preparing data for analysis.
- Analysis and modeling: Using statistical techniques and machine learning to analyze data.
- Interpretation: Translating data findings into actionable business insights.
Data scientists also specialize in data visualization and digital transformation. They convert complex data into easily understandable visuals, helping stakeholders make informed decisions.
Moreover, their work extends to ensuring data governance and security. They help maintain compliance with data privacy regulations and safeguard sensitive information.
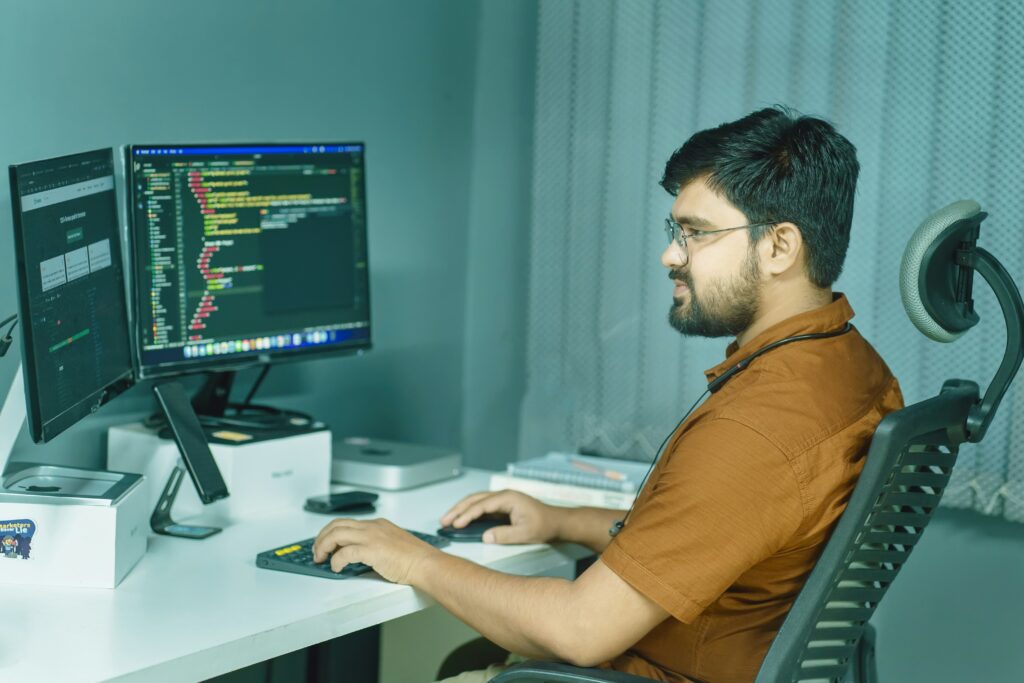
Data scientist can transform your organization’s approach to decision-making. For example, data scientists can forecast market trends ещ enablу your company to adapt strategies proactively.
Additionally, they can improve efficiency within operations. With predictive analysis, they can streamline processes and optimize resources.
Data Scientist vs. Data Analyst vs. Data Engineer
While these roles might seem similar, they have distinct functions.
- Data scientist focuses on modeling and interpreting complex data. They are the strategists who guide company decisions.
- Data analyst primarily examines data to answer specific questions. Their work is often more exploratory and doesn’t usually require advanced modeling.
- Data engineers, on the other hand, are the builders. They construct and manage the infrastructure for data generation, ensuring that data flows smoothly and is accessible for analysis.
Sign #1: Your Data is Overwhelming Your Current Capabilities
Today’s businesses collect a lot of data daily. This data comes from diverse sources, both internal and external, and is often complex. Managing it effectively requires expertise in data science job roles and the right frameworks to extract meaningful insights.
If your current team struggles to process this data effectively, it might be time for a change. The data that can’t be analyzed might just as well not exist. Unstructured data, in particular, can be challenging, requiring advanced business intelligence tools for interpretation.
Consider also the growing speed at which data flows into your organization. Real-time analytics requires not just processing power but sophisticated skills, often seen in data science professionals who specialize in extracting insights from complex datasets.
Here are some signs your data might be overwhelming you:
- Increased data volume: Your data is growing faster than it can be analyzed.
- Diverse data sources: Handling multiple types of data from different platforms.
- Lack of insights: Difficulty in turning raw data into actionable insights.
An overwhelmed system leads to missed opportunities. Slow response times and outdated reports can affect strategic decision-makers, impacting business growth.
Furthermore, the more data piles up, the more daunting the task becomes. This complexity might necessitate advanced data management methods beyond your current team’s skills, including predictive analytics and machine learning models used in Kaggle competitions.
When data goes unanalyzed, critical business insights are missed. This could mean overlooking market trends or customer preferences that could be identified using regression models.
Competitors could then gain an edge by identifying these insights first. They might launch campaigns or products that attract customers your data hinted at.
Moreover, unutilized data can lead to inefficiencies in operations. Without insights, improvements in metrics related to productivity or efficiency might go unnoticed, costing your business time and money.
Sign #2: You Miss Insights That Could Scale Your Business
Every business decision hinges on available information. When critical insights are missing, opportunities slip through the cracks, often unnoticed.
Data-driven insights offer a powerful way to identify new market trends. They can reveal customer preferences, paving the way for innovation in both startups and established businesses.
Companies harnessing these insights often enjoy a competitive advantage. Their actions are not based on guesswork but on solid hypotheses tested with real-world data.
Missing insights manifest in several ways:
- Stagnant product development: Lacking insights into what consumers want.
- Inefficient marketing campaigns: Not targeting the right audiences.
- Operational bottlenecks: Failing to streamline processes due to lack of data.
Accessing and interpreting data insights requires specialized data science skills. It’s about seeing patterns or opportunities that aren’t evident to the untrained eye. For example, many professionals in the field of data science leverage platforms like Kaggle to improve their analytical capabilities and improve forecasting accuracy.
Additionally, missing insights can lead to poor customer experiences. Without personalization based on data insights, businesses may fail to connect effectively.
Overall, leveraging data insights can unlock unexplored revenue paths. It allows a company to adapt swiftly and efficiently to market changes, often using artificial intelligence solutions integrated through APIs for seamless automation.
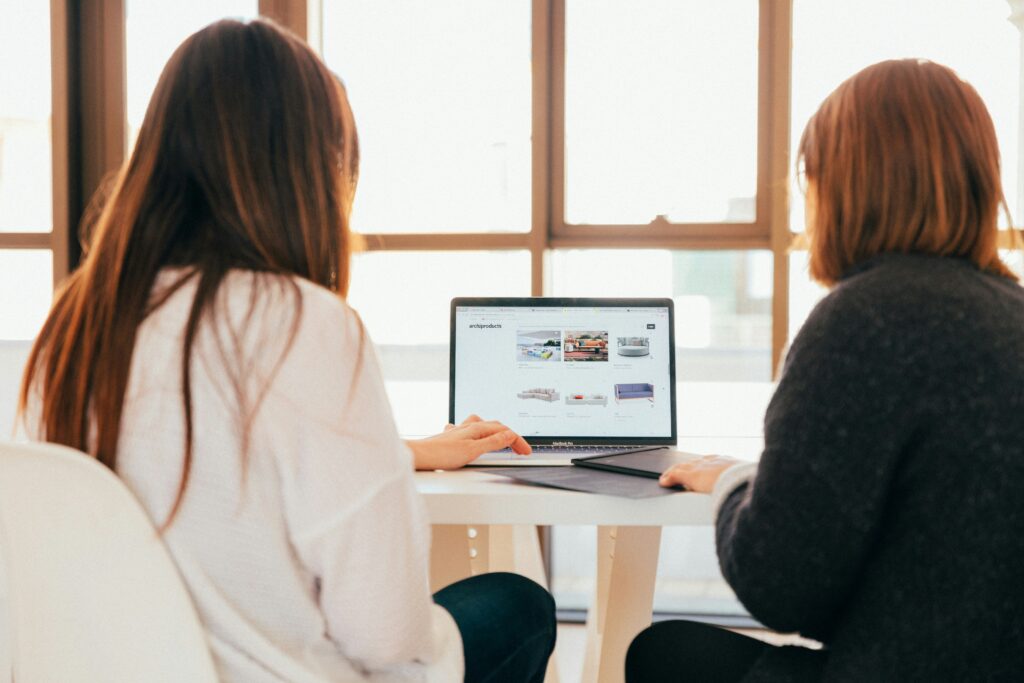
For instance, take a retail company that turned to data science projects for increased personalization. By analyzing consumer purchasing behavior, they optimize their marketing strategies effectively. Their efforts led to an uptick in customer engagement and sales growth. Predictive models forecasted demand, optimizing inventory and reducing waste.
Another example is a logistics firm optimizing routes using business intelligence and real-time data analytics. Hadoop-based processing helped them cut delivery times and slash fuel costs.
Both examples illustrate how turning big data into insights can transform business practices. The potential return on investment from a data scientist’s expertise is substantial, particularly for organizations leveraging Microsoft’s cloud-based analytics solutions.
Sign #3: Falling Behind Competitors in Data Utilization
Falling behind in data utilization means slower responses to industry changes. It can also result in missed opportunities that your competitors seize.
Many companies recognize the need to harness data for decision-making. This is especially true in sectors like finance, healthcare, and software engineering.
Here are some signs your competitors are using data better:
- Quicker market adaptation: Competitors launch products faster due to insights.
- Improved customer engagement: They personalize interactions efficiently.
- Operational excellence: Processes optimized using data lead to cost savings.
A critical advantage of data science is its predictive power. By predicting trends, businesses can prepare for shifts before they happen, utilizing techniques such as regression analysis for forecasting.
Moreover, a proactive data strategy fosters innovation. It allows companies to take calculated risks with a higher chance of success, particularly in industries where Java-based analytics frameworks are widely adopted.
Staying competitive means continually refining your data science job applications—not just gathering data, but analyzing and applying insights swiftly.
Sign #4: Complex Data Problems Need Advanced Analytics
Many businesses face growing data complexity. Simple analysis no longer suffices for these data challenges.
In such cases, advanced analytics becomes indispensable. It enables businesses to solve intricate issues efficiently, often requiring expertise in Kaggle competitions or academic training, such as a master’s degree in data science.
Without advanced analytics, data remains unstructured and unused, preventing businesses from gaining valuable insights. Companies in finance, healthcare, and manufacturing increasingly rely on predictive analytics and AI-driven initiatives.
Here are some benefits of advanced analytics:
- Predictive maintenance: Avoid equipment failures in manufacturing by anticipating issues.
- Fraud detection: Identify suspicious patterns in financial transactions automatically.
- Customer segmentation: Divide customers into meaningful groups for targeted marketing.
The use of machine learning algorithms is vital here. They can process a lot of data rapidly and detect hypotheses that would be impossible for human analysts to spot.
Sign #5: Your Team is Overwhelmed and Lacks Specialized Skills
An overburdened team can show the need for specialized talent. When teams handle too much, productivity and morale drop.
In data-rich environments, this problem is more pronounced. The absence of expertise can prevent the effective use of data management tools.
Data scientists excel where others struggle. They possess unique skills essential for interpreting complex datasets and integrating Hadoop-based solutions for large-scale processing.
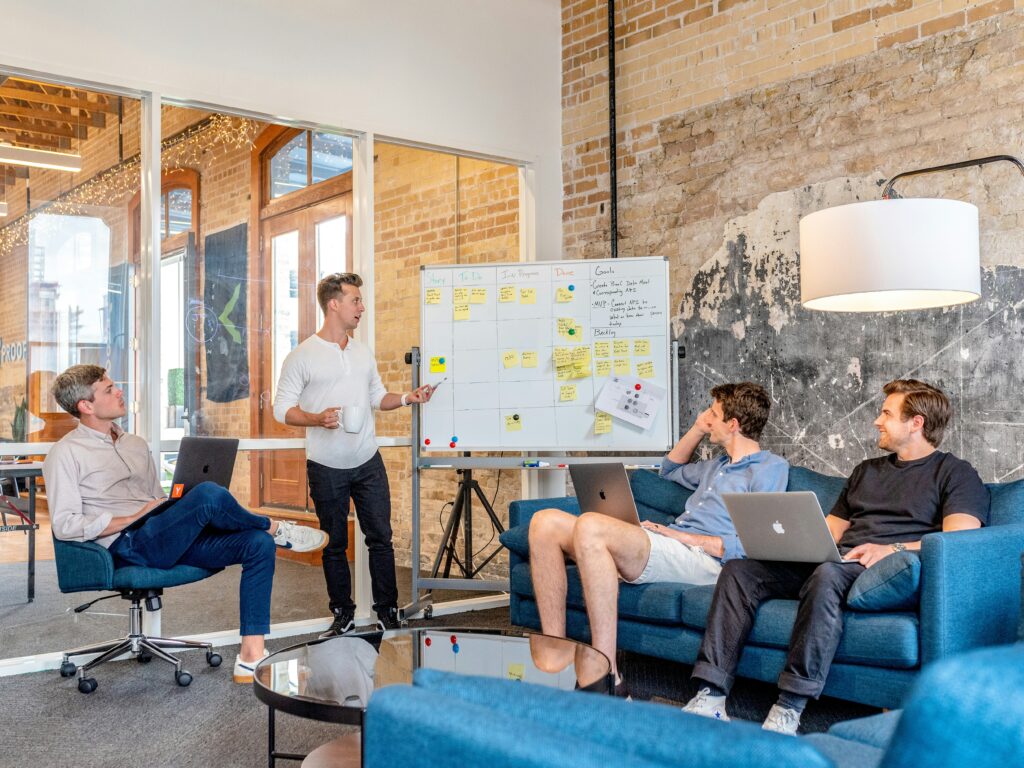
Signs that your team might need extra help:
- Inefficiencies: Are routine tasks taking too long?
- Missed deadlines: Is your team constantly behind schedule?
- Lack of creativity: Are novel ideas diminishing over time?
A statistician or a data scientist brings a fresh perspective. Their insights can spark innovation within your organization and help build data-driven APIs for real-time decision-making.
Without specialized skills, businesses risk stagnation. Resisting change can lead to missed opportunities and competitive loss.
The Cost of Not Hiring a Data Scientist
Neglecting the need for a good data scientist can lead to significant setbacks. Without skilled data science professionals, businesses miss out on vital insights.
Lost revenue and reduced competitive edge often follow. Data science projects hold the key to understanding market trends and consumer behavior, making the role of a data scientist or programmer critical in today’s economy.
Ignoring the potential of data is a luxury few can afford. The strategic use of data is a clear path to sustained growth.
How to Assess and Hire the Right Data Scientist
Hiring the right data scientist involves a clear understanding of your business needs. First, define the specific problems you want to solve with data.
A thorough job description sets the fundamentals like required skill set and project responsibilities clearly.
When evaluating candidates, look for a blend of technical prowess and practical experience. Knowledge of machine learning, programming, and statistics is essential. Soft skills shouldn’t be overlooked. A strong ability to communicate insights and collaborate with non-technical teams is crucial.
Conducting technical interviews helps gauge a candidate’s problem-solving skills. Real-world data scenarios can reveal their capability.
Prepare to offer competitive compensation, reflecting the high demand for data science roles. Attractive packages help attract top talent.
Here are some key factors to assess:
- Technical skills: Expertise in analytics, algorithms, and data visualization tools.
- Experience: Hands-on experience in solving business problems with data.
- Communication: Ability to convey complex insights in simple terms.
- Cultural fit: Alignment with your company values and team dynamics.
- Problem-solving: Innovative approaches to addressing data challenges.
Selecting the right candidate involves more than just skills. Their ability to fit within your team’s culture affects success.
Qualifications, Skills, and Cultural Fit
Qualifications are critical when assessing data science candidates. Advanced degrees in related fields often denote technical expertise.
Look for skills in programming languages like Python or R. Statistical analysis and machine learning knowledge are also vital.
Cultural fit is equally important. A candidate should share your company’s values and work well with existing teams.
Consider their past experiences and how they approached challenges. This helps determine if they align with your business needs.
Teamwork and adaptability are key traits. They enable data scientists to integrate smoothly and contribute effectively.
5 Quick Tips for the Recruitment Process
- Streamline your recruitment by defining clear criteria from the start. This helps focus on candidates who meet your needs.
- Involve multiple stakeholders in the interview process. Different perspectives ensure a well-rounded assessment.
- Offer practical tests to see how candidates handle real data scenarios. These tests reveal their analytical thought process.
- Utilize networking on LinkedIn and specialized job boards to find talent. Tech meetups and conferences are also great places to scout.
- Consider hiring consultants for interim solutions. They can address immediate data challenges while you find a full-time hire.
Conclusion
The business landscape is rapidly evolving, with data at its core. To remain competitive, leveraging data science is no longer optional. Understanding your data can uncover new opportunities and drive strategic decisions.
Hiring a skilled data scientist is vital to unlocking these possibilities. They bring not just technical skills but also insights that can transform your business processes. Don’t wait until you’re struggling with data challenges—act now. Embrace the power of data science to enhance growth, innovation, and market leadership.
FAQ
How to hire a data scientist quickly?
To hire a data scientist quickly, start by clearly defining the skills and qualifications you need. Use specialized job boards and networking sites to reach potential candidates. Streamline your recruitment process by involving key stakeholders in decision-making.
Conduct practical assessments to evaluate candidates efficiently. Use Generation AI to access a larger talent pool and identify top candidates. Ensure your job description is compelling to attract interest. Finally, maintain open communication with candidates to expedite the process.
How much does it cost to hire a data scientist?
The cost to hire a data scientist varies greatly based on experience and location. Entry-level data scientists may earn between $70,000 and $90,000 annually, while experienced professionals can demand $120,000 to $200,000 or more. Additionally, factors such as industry and the complexity of the job also influence salary ranges.
Consider recruiting from areas with lower costs of living to manage expenses. Don’t forget to include recruitment fees and potential onboarding costs. Offering competitive compensation is essential to attract top talent. Budgeting for ongoing training and development should also be considered.
How long does it take to hire a data scientist?
Typically, the hiring process for a data scientist can range from a few weeks to several months. However, with Generation AI, it takes up to 2 days to hire the best-fit professional for your team. This expedited process is achieved through advanced algorithms that match candidates with your needs. The efficiency of AI tools can significantly reduce time-to-hire.
Streamlining your selection process and focusing on essential skills further enhances speed. An urgent need for data science talent can thus be met promptly. Quick hiring doesn’t compromise the quality of the candidate, ensuring you find the right expertise.